ML Ops and Architecture
Experience the transformative power of our ML Ops and Architecture services. By partnering with us, you'll benefit from streamlined machine learning workflows, scalable infrastructure, and optimized performance. Harness the full potential of ML with efficient operations, cost savings, and enhanced governance. Trust our expertise to elevate your ML initiatives and drive tangible business outcomes.
Key Benefits
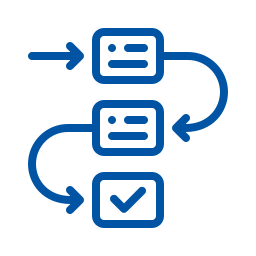
Streamlined Machine Learning Workflows
Optimize your machine learning workflows with our ML Ops expertise. From data preprocessing to model deployment, our streamlined processes ensure efficiency, collaboration, and faster time-to-market for your ML projects.
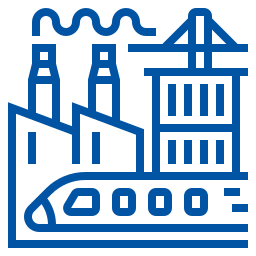
Scalable and Reliable Infrastructure
Leverage our expertise in designing and implementing scalable and reliable ML infrastructure. We provide robust data pipelines, scalable storage solutions, and efficient computation resources, ensuring seamless operations as your data volumes and models grow.
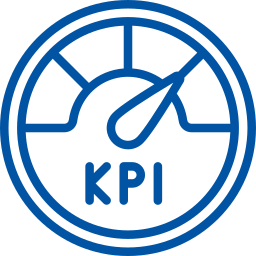
Enhanced Model Performance and Monitoring
Drive better results with optimized model performance and comprehensive monitoring. Our ML Ops practices include continuous integration and deployment (CI/CD), automated model training, and monitoring systems for detecting anomalies, ensuring optimal model performance.
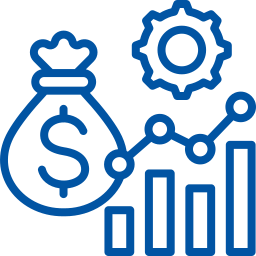
Cost Optimization
Optimize costs associated with ML deployments. Our ML Ops strategies help you achieve efficient resource allocation, workload management, and automation, reducing infrastructure costs and maximizing your return on investment (ROI).
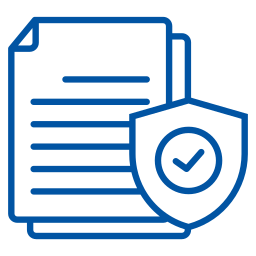
Governance and Compliance
Ensure governance and compliance in your ML workflows. We implement security measures, data privacy controls, and regulatory compliance frameworks, safeguarding sensitive data and ensuring adherence to industry standards.
Machine Learning Operations (MLOps) and Architecture offers unparalleled efficiency and speed in the development, testing, and deployment of ML models. By implementing MLOps, businesses adopt a systematic approach that significantly accelerates the development cycle. MLOps also provides better collaboration between teams, unifying the objectives of data scientists and IT professionals for a streamlined workflow. Enhanced model performance and scalability are notable benefits, with the architecture designed to handle large volumes of data and complex computations. Furthermore, MLOps promotes reproducibility and auditability in the model development process, which is crucial for regulatory compliance and maintaining the quality and reliability of the models. With continuous integration, delivery, and learning in place, businesses can adapt quickly to changes and continuously improve their models over time, gaining a competitive edge in their industry.